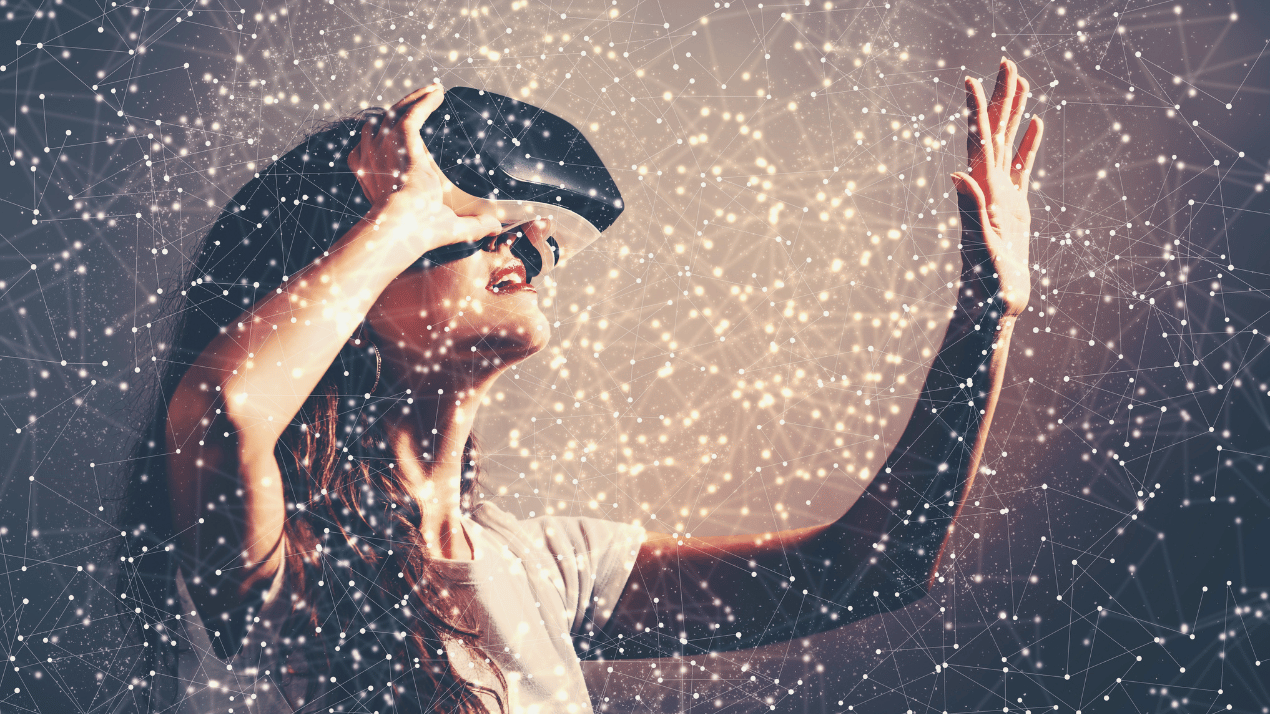
Event details
Abstract:
Nowadays, there are applications where data is best modeled not as persistent tables, but rather as transient data streams.
In this talk, we discuss the limitations of current machine learning and data mining algorithms and the fundamental issues in learning in dynamic environments like learning decision models that evolve over time, learning and forgetting, concept drift, and change detection. Data streams are characterized by huge amounts of data that introduce new constraints in the design of learning algorithms: limited computational resources in terms of memory, processing time, and CPU power.
In this talk, we present some illustrative algorithms designed to take these constraints into account. We identify the main issues and current challenges that emerge in learning from data streams and present open research lines for further development.
About the Association for Computing Machinery (ACM)
ACM, the world's largest educational and scientific computing society, delivers resources that advance computing as a science and a profession. ACM provides the computing field's premier Digital Library and serves its members and the computing profession with leading-edge publications, conferences, and career resources.
Speaker
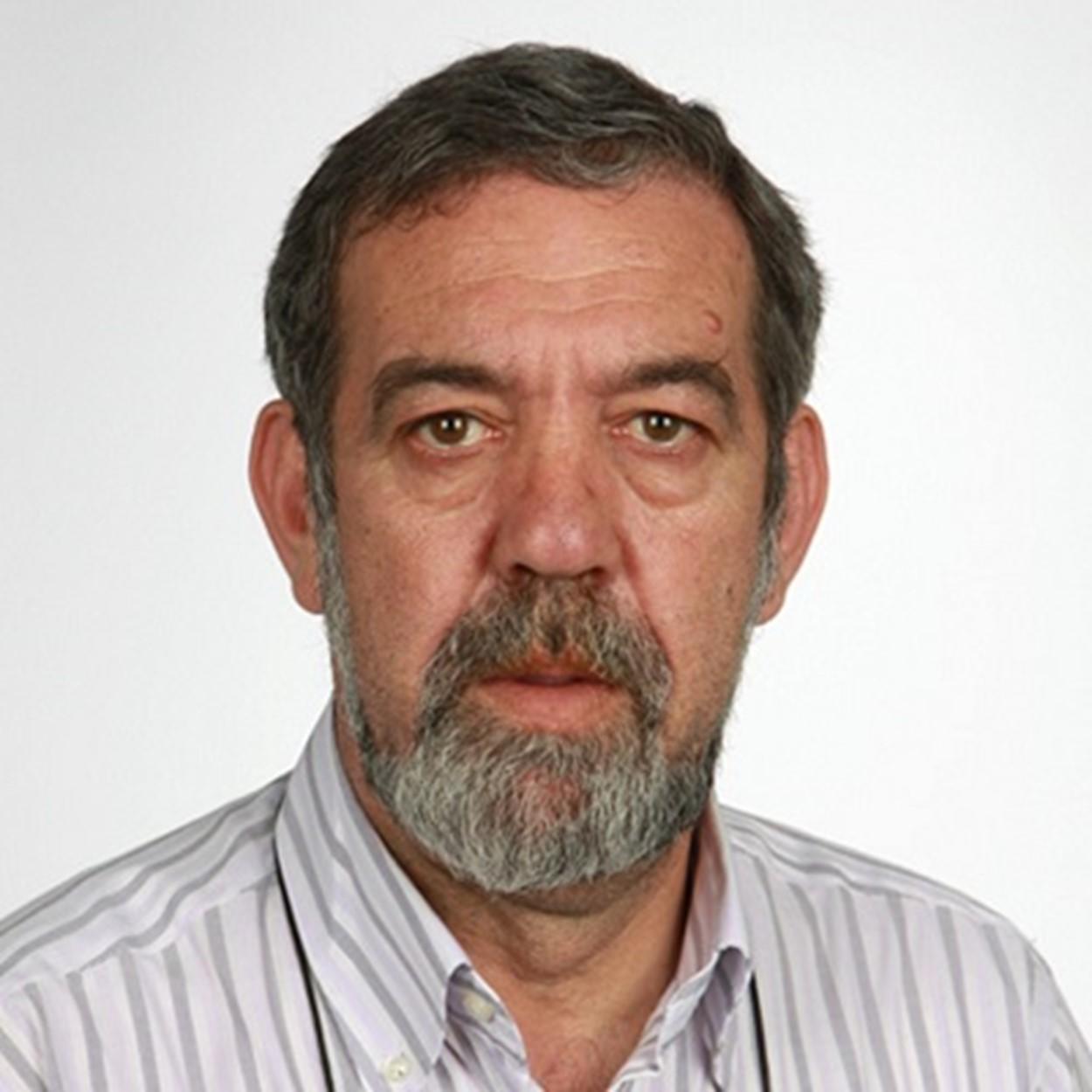
João Gama
Full Professor at the School of Economics, University of Porto, Portugal
João Gama received his Ph.D. in Computer Science from the University of Porto in 2000. He is EurIA Fellow, IEEE Fellow, Fellow of the Asia-Pacific AI Association, and member of the board of directors of the LIAAD, a group belonging to INESC TEC. His h-index on Google Scholar is 67. He is an Editor of several top-level Machine Learning and Data Mining journals. He is ACM Distinguish Speaker. He served as Program Chair of ECMLPKDD 2005, DS09, ADMA09, EPIA 2017, DSAA 2017, served as Conference Chair of IDA 2011, ECMLPKDD 2015, DSAA’2021, and a series of Workshops on KDDS and Knowledge Discovery from Sensor Data with ACM SIGKDD, and ACM SIGAPP.
His main research interests are in knowledge discovery from data streams, evolving data, probabilistic reasoning, and causality. He published more than 300 reviewed papers in journals and major conferences. He has an extensive list of publications in data stream learning.
Students, professors, industry, general public